When we assess someone with a score — whether it’s a university exam or an IQ test or an Olympic diving result — the number only makes sense when it’s put in context.
In the United States, students are used to being graded on a 100-point scale. It’s generally accepted that a 90 or above is a good score; below 60 is catastrophic. When I began studying in Europe, I had to adjust from a 100-point scale to a 20-point scale. It took a little while to realize why my classmates were so happy about getting a 17 on an assignment!
Context gives numbers meaning. For a score to convey information — and for it to become actionable for the person receiving it — we need to understand the scale being used, as well as reference points for different score levels.
When Workera assesses users, it measures their knowledge in an individual domain on a 300-point scale. In this article, we’ll take a look at why we use this range of numbers; how users should interpret their results; and what actions they should take after completing an assessment.
Breaking down Workera’s skill levels
Instead of thinking about these scores on the complete scale of 1–300, it may be more useful to think of them as three sets of 100-point scales stacked on top of each other:
- Beginning (1–100): Able to recognize and understand simple concepts and applications in a domain
- Developing (101–200): Able to evaluate and interpret problems, and discuss solutions to them
- Accomplished (201–300): Able to design, develop, and build solutions to problems
Which of these three scales you fall in will determine which content you should study and what actions you should take. There is a meaningful difference when you transition from one level to the next: there are different skills associated with the beginning level than with developing and accomplished. Once your score improves from 100 to 101, the platform will begin evaluating you on a different set of skills — the knowledge and abilities it associates with “developing” in that domain.
Your score on the 300-point scale is useful in a broad sense: it will indicate your general ability level and give you a sense of where you need to improve. However, this score means more — and should be taken more seriously — when it’s connected to benchmarks and organizational goals. Knowing that your score in AI Explainability is 130 doesn’t tell you much; but if you know that the enterprise benchmark for AI Explainability is 150, you suddenly have much more context on where you stand and how much you need to improve. Some organizations might set team-wide goals for certain domains: if your entire team is expected to reach 225, you will have a better understanding of what is required to close the gap.
For Workera users, orienting around industry benchmarks or organizational goals will be more productive than simply chasing progress or a perfect, 300-point score. Every employee’s role will consist of multiple domains, and no employee should be expected to achieve an exceptionally high score in every domain. An outstanding employee may be Accomplished in eight domains while still Developing in a few others.
How Workera produces a score
Imagine you’ve completed one of Workera’s computerized adaptive tests (CAT), and you discover that you’re Developing (114) in AI Fairness. How did the platform produce such a precise number?
Every domain consists of dozens or even hundreds of individual, highly granular skills; for example, our SQL Foundations domain comprises 64 specific skills. In every domain, those skills are ordered according to difficulty. In the SQL Foundations example, the algorithm understands which skill is the 15th most difficult, which is 38th, which is 64th, and so on.
The algorithm begins a CAT with one question and then will produce an easier or more difficult question based on the user’s answers: the questions will steadily increase in difficulty with more correct answers, and will decrease in difficulty in response to wrong answers.
After 20 questions, the algorithm is capable of producing a score on the 300-point scale and placing them within one of the three levels. This score isn’t just determined using the user’s answers; the algorithm also draws correlations and conclusions based on anonymized historical data from previous users and assessments.
Once the user has completed their initial assessment, the platform then creates a learning path that is calibrated and tailored to the user’s current ability level. This learning path will serve the user the right content in the right order, allowing them to upskill on the information they need without wasting time on irrelevant content.
What matters most: learning velocity
When a user conducts a first assessment on Workera, or reassesses after a period of learning, they are gaining a snapshot of their ability level at one moment in time. That information can be useful, but other metrics are more important for both individual and team development.
For a skills-based organization, the most important metric is learning velocity: how many points were you able to add to your score between your first assessment and reassessment in a domain? Adding 5–10 points per week is considered standard; anything above 10 is best in class. Bear in mind that employees can typically upskill in more than one domain at a time; a typical data employee will upskill in three to four domains in parallel.
Your learning velocity determines how quickly your organization will be able to innovate; how prepared it will be to adapt to new technologies; and how well it can fend off competitors. One organization may have employees that are stronger than their competitors on their initial assessment. But over time, if another less experienced organization is capable of learning faster, they will eventually catch up and overtake the incumbent leader.
To learn more about how Workera measures skill levels, read our recent blog post about measurement instruments — part of The Leader’s Guide to Skills-based Organizations.
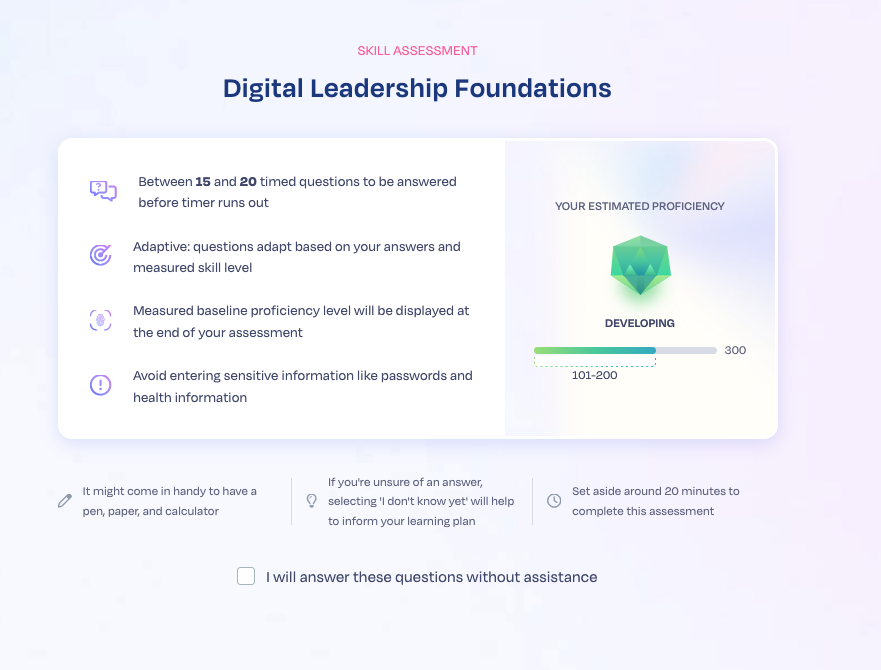